What if we could build personalized digital twins that can identify anomalous health events that lead to cancer, suggest lifestyle changes to avoid high-risk states, and, when needed, instigate early diagnostic steps? what if the clinician and the cancer patient could jointly explore personalized risks, benefits and side effects and choose the best treatment plan using the patient's digital twin?
These and other questions were explored in an intense 5-day Idea Lab organized by the National Cancer Institute, the Department of Energy and the Frederick National Labs for Cancer Research.
Imagine this scenario
On the day a person hears of his/her cancer diagnosis, a digital twin of the patient is instantiated by the clinical team. Over time, multi-modal, individualized data from genetic, environment and social factors are fed to the digital twin to create an accurate representation of the current state of the cancer patient. Clinicians then use High performance computing to simulate all treatment options on the digital twin, thus completely avoiding any trial and error experiments on the patient. Together, clinician and the patient explore risks, benefits and side effects and collaborate to choose the best treatment pathway in a data-driven manner. The digital twin continues to be update with new data from patient on outcomes, resistance to treatment and cancer progression to be ready and available for new simulations during the next visit.
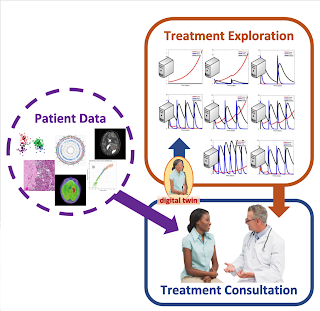 |
Envisioning Computational Innovations for Cancer Challenges (ECICC) Micro lab, June 2019 |
What would such a cancer digital twin enable?
If done right, with the aid of digital twins, clinicians will be able to predict adverse events, progression-free, and overall survival in the twin models and change the course of treatment or guidance for patients accordingly. The digital twin will provide a multitude of benefits to patients by predicting future state (recurrence, resistance to treatment, etc), provide the probability that the patient now has an anomalous molecular state so they can seek clinical consultation, and inform the patient about appropriate lifestyle or diet changes to reduce risk of cancer incidence (if healthy) or improve outcomes (if cancer has already been diagnosed). Using digital twins, researchers may be able to rapidly simulate Randomized Controlled Trials (RCTs) using case and control digital twins which can help save time and resources.
What are some of the key challenges?
In order to fully realize the potential of cancer digital twins, multiple barriers have to be overcome including, but not limited to, availability of coordinated data across multiple scales for a patient, guidelines for virtual cancer care pathways, and multi-scale HPC (High Performance Computing)-driven analysis of data. Regulatory landscape for use of digital twins in care pathways and at homes will have to be assessed carefully.
Potential larger impact:
A multitude of efforts are underway to advance precision oncology including large, multi-scale data collection programs such as TCGA (The Cancer Genome Atlas), CPTAC (Clinical Proteomic Tumor Analysis Consortium), HTAN (Human Tumor Atlas Network), and novel trial designs that match a patient to the most appropriate therapy based on their tumor molecular profile, e.g. I-SPY, NCI-MATCH (Molecular Analysis for Therapy Choice), TAPUR (Targeted Agent and Profiling Utilization Registry), SMMART (Serial Measurements of Molecular and Architectural Responses to Therapy). Building on these initiatives, the cancer digital twin program can fully utilize these investments made in datasets and infrastructure to achieve the true vision of precision oncology. As AI and mechanistic models improve and more data become available at individual, study- and population-levels, this would enable personalized benefit from different diagnostic and treatment modalities through the incorporation of genetic, environmental, and social factors to predict individual trajectory for each and every patient.
You can watch this space for further updates on this initiate.
Comments
Post a Comment